By Selim Nahas
Factory automation increasingly depends on our ability to make complex decisions rapidly, but many semiconductor fabrication processes have exceeded the cognitive capabilities of humans to troubleshoot anomalies and assess all relevant variables. Thus, automation has become a key strategy used to address these challenges.
At Applied Materials, a group of automation engineers is exploring new methods to better bridge the human-machine gap, including the use of AI-based techniques in inline statistical process control (SPC) for wafer measurements. SPC is the process by which various data points are collected for each step of the manufacturing process, making it possible to determine whether a given step was completed correctly.
The SPC technology development activities are taking place within the Applied SmartFactory™ Rapid Response Inline Measurement platform. These are aimed at providing automated guidance for root cause analysis, standardizing the approach to resolve SPC violations, and providing integrated troubleshooting workflows to reduce human variability in semiconductor manufacturing.
This article provides an overview of the goals, requirements and strategies involved with new and AI-based SPC methods.
Evolving AI
AI is an evolving discipline and will likely be applied first in existing facilities, to demonstrate its anticipated agility and functionality. This is because greenfield facilities won’t use AI without evidence of its performance, which can be more effectively assessed in a functioning facility. AI-based techniques are so new they do not yet have clear key performance indicators (KPIs) to demonstrate a proven track record, nor is there a clear idea of how to effectively manage AI through its learning lifecycle on a factory-wide basis (figure 1).
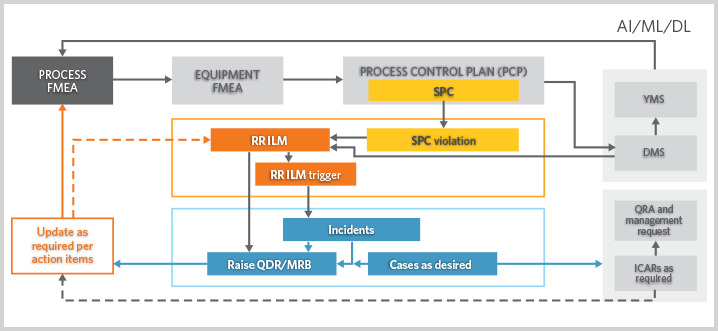
Figure 1. Existing fab infrastructure will serve to close the loop on validation and learning for AI functions. (RR ILM is the Applied SmartFactory Rapid Response platform solution.)
Another constraint comes from the negotiated performance expectations of the customer. Performance-tospecification requirements are contractual obligations that are difficult to renegotiate or alter. In time, these standards will evolve, but this change will be progressive.
An additional aspect of the evolving AI landscape is that the initial acceptance of such systems needs to be encouraged. Factory operators must gain confidence in the system, recognizing that the goal is not “hands-off” factory operation but rather automation-assisted manufacturing decisions that make speed, repeatability and accuracy the primary focus.
Initial Applications
The use of AI in fab operations is expected to result in high degrees of repeatability, consistency, and greater speed of decision-making, ultimately leading to higher-quality decisions that deliver better performance (figure 2).
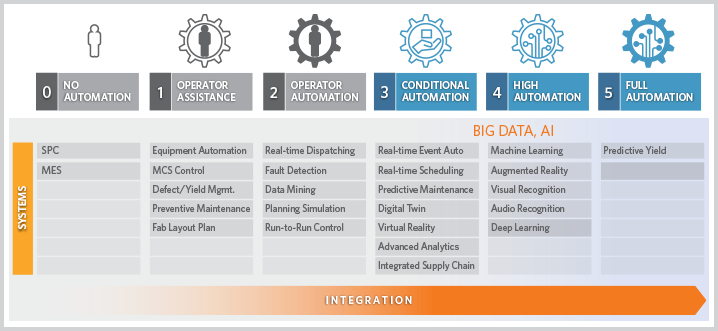
Figure 2. The industry will progressively adopt big data and AI functions. (Source: MIT)
Anomaly handling is a good place to initiate AI implementation in a fab because a significant portion of material loss in the form of scrap and delays is attributable to human error. Anomaly handling can effectively be considered for either the process- or equipment level. Therefore, this article focuses on inline SPC for both process- and equipment performance in a front-end semiconductor facility.
The last few years have seen an evolution in the resources available to assist fab personnel in handling the inline SPC disposition problem, beginning with paper-based out-of-control action plans (OCAPs) and progressively migrating to integrated linear systems that facilitate step-by-step "how-to" instructions to the operator. This approach will soon prove insufficient, for several reasons. First, process dependencies and variables are not understood equally by everyone. Also, no empirical metric exists to tell users what constitutes an effect on the current process coming from upstream. And finally, when an anomaly is detected, it is often difficult to know the extent to which it impacts the current processes.
Key questions, therefore, are how to understand and quantify the contribution of an anomaly to process variance, and how to enable the system to quickly verify that a proposed solution is the right one? In today’s fabs, most SPC systems are focused on lot-to-lot and wafer-to-wafer variation reduction. There is an insufficient focus on within-wafer variation, which also needs to become one of the primary KPIs of AI systems.
In a previous article[1] we explored the use of existing data to visualize underlying problems. While many fabs rely on mean statistics to govern process quality, site-level data is a more effective metric for judging wafer-to-wafer and within-wafer variations. The challenge comes from the number of elements to monitor. Most fabs suffer from an excessive number of SPC charts, and the number of charts required to monitor site-level statistics would add to the burden.
Process- And Equipment Failure Modes
One approach is to recognize that we must employ both process- and equipment failure mode and effects analysis (FMEA) in our proposed solution, because to be effective the AI system must distinguish between process- and equipment anomalies at runtime. Such a system cannot be set up manually, because a logic fab typically contains 750 to 2,000 tools used to manufacture chips with up to 60 layers, including up to 14 critical layers.
Many things can go wrong, given the extensive interdependency within a device’s critical layers, as 300mm nodes are heavily dependent on defect-free alignment of several layers to assure proper feature dimensions and characteristics. Wafer topography and chemistry can affect deposition quality, and wafer planarization may be affected as a result. Lithography can ultimately be impacted in the form of alignment and focus problems on the desired registration marks. Finally, etch can inherit these variations and not be able to contend with them.
When the process FMEA is defined, we will find that each of these upstream processes has multiple measurements for each parameter, and each measurement lends insight into wafer quality. For operators, the number of permutations they would have to evaluate would require not only an understanding of each measurement’s effect but also the severity of its contribution to the observed variation. This is impractical.
The equipment FMEA, meanwhile, provides insight into uniformity problems across the wafer because it monitors a select set of variables attempting to sort equipment problems from process problems. This includes the equipment of upstream processes.
The fundamental difference between the two FMEA analyses is that in one case a tool’s ability to uniformly process is monitored, and in the other case the performance of each wafer coming from multiple process adjustments is evaluated, along with calibration variation. For example, with deposition or etch rates, the analysis tries to determine whether the equipment is delivering the intended rate. If not, deriving a site-level statistic to monitor for rates of change can provide insight that the automation system can use to make a triage decision between process-and equipment problems.
In instances where both process-and equipment anomalies are prevalent, the challenge becomes considerably greater. When erroneous decisions are made the need is to rapidly recognize and rectify them. It is anticipated that an AI system should fundamentally outperform a human in this regard. This will involve the use of some new KPIs that are not currently industry standards, such as repeat violation rates and sigma standards on site-level calculated parameters (figure 3).
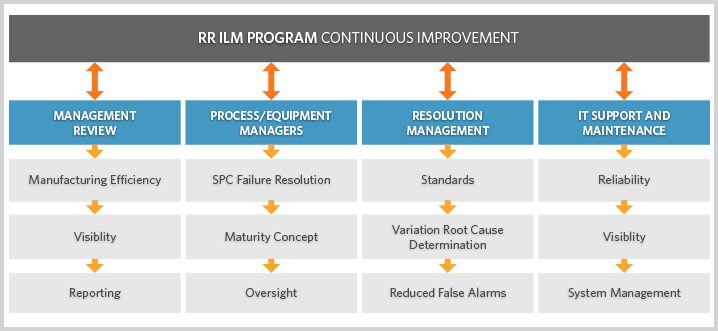
Figure 3. The evolution of performance tracking. Each of the four segments will become dashboard-driven with existing and new KPIs. (RR ILM is the Applied SmartFactory Rapid Response platform solution.)
The user must first identify all the known information regarding the samples that have violated limits. These include the path through the various processes, the recipes, the product, and the resulting variation or shifts on the wafer.
Then, the user must make several quick assessments to begin the troubleshooting process. The first is to understand the severity of the failure, and the process equipment and wafers impacted by the condition. Depending on the severity, the process can be halted by recipe, or by chamber and equipment. Next, the user must evaluate the validity of the measurement, and ensure that the right number of raw data points are available from a process that has historically been reliable and repeatable.
Finally, the user must determine whether the failure is coming from a prior process or an equipment drift or both.
Outlining the variables to these questions enables us to quickly create an automation roadmap. First, a process FMEA can be built into the behavior of the system to allow us to isolate the incoming failures. Then, an equipment FMEA can be built into the system. An analysis of variance (ANOVA) can then be developed to determine which is contributing more to process variation.
Measurements will be made wafer-to-wafer and within-wafer, and most important of all, will include site-level measurements from wafer to wafer. The process FMEA will ultimately sort the variation sources against the fleet and consider tool and chamber matching as a set of variables. It will convert everything into a go/no-go action when a knob is not available to tune a variance.
Closing the Loop
As previously mentioned, a learning system must be built that will ultimately update our understanding of the process- and equipment FMEAs and evaluate the effectiveness of the decisions. One key metric will come from the fab’s yield management system (YMS), while another key metric will come from a new set of KPIs centered around within–wafer variance, which will accompany the already existing KPIs.
These new KPIs are typically not negotiated with customers, and each manufacturing step will have a new set that becomes available with AI systems. The standard-quality KPIs will persist, but they will be augmented by new ones such as repeat violation rates, time to decision, quality of decision and others. All process- and equipment learning will update the FMEA built into the AI system.
Such changes have tremendous implications for fabs. Consider that a fab with 20,000 wafer starts per month (WSPM) typically experiences more than 2,500 events per month. Out-of-spec (OOS) events typically take between 25 to 40 minutes each to troubleshoot, and most of these OOS conditions become scrap when a rework is not possible. Out-of-control (OOC) events also impact processes depending on a fab’s practices. And OOC events frequently become OOS events if not kept in check.
The AI system will provide a speed-of-use component that will reduce OOS resolution time to under four minutes when an operator needs to confirm the results recommended by the system, and will reduce it even further under a full-automation scenario. This will constitute a major departure from current standards. Faster and higher-quality decisions translate into fewer repeat violations, further reducing interruptions.
In such a fab, the improvements offered by AI-based SPC systems are in the speed and quality of decisions. These improvements may translate into more than 600 hours of process time recovered per month for production, along with a further reduction of scrap of approximately 10% from current rates.
Within-wafer variation reduction may also translate into yield gains at advanced nodes, though this has yet to be quantified. The same line of reasoning can be employed to other production process anomalies such as fault detection and electrical testing.
Conclusion
Developing AI-based next-generation quality systems will enable customers to make higher-quality decisions in a shorter amount of time by either fully automating decision-making or augmenting human decision-making.
Successfully implementing these systems will require deep industry experience, a creative and innovative mindset, and a strong technological foundation, such as Applied’s expansive SmartFactory portfolio of integrated capabilities. At its core, this portfolio utilizes industry-leading, best-of-breed technologies combined with industry-standard resources, collaborative design and methodical research to help customers propel quality to new levels.